Ebook: The 10 Rules of Reliable Data Science
We've worked on 100+ data science projects with dozens of organizations, from new startups to large foundations and Fortune 50 companies. We've seen thousands of data science projects submitted to our data science competitions by practitioners and researchers.
As the field develops, it is becoming increasingly important to organize data science work so that it is easy to understand, reproduce, and build upon. Here's what we've learned about the best practices—and the perils—for data science workflows.
Complete the form to download our free ebook
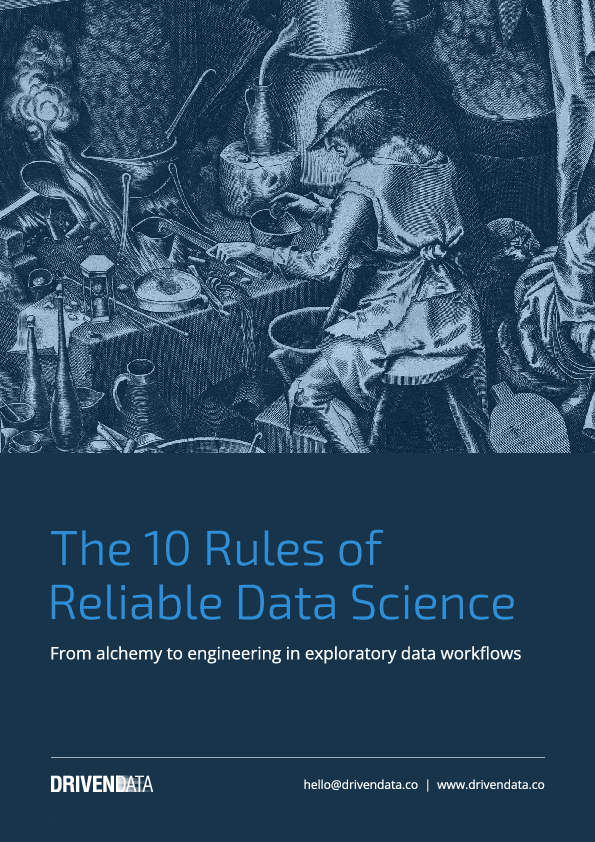
Watch the video
Co-founder Isaac Slavitt presents some key points from the ebook at PyData Global 2022.
Abstract: Data science as a professional discipline is still in its infancy, and our field lacks widespread technical norms around project organization, collaboration, and reproducibility. This is painful both for practitioners and their end users because disorganized analysis is bad analysis, and bad analysis costs money and wastes time. This talk presents ten principles for correct and reproducible data science inheriting from software engineering's seven decades of hard-earned lessons as well as numerous experiences with data science teams at organizations of all sizes. We motivate these principles by looking at some hard truths about data science “in the wild.”