The Community Spotlight celebrates the diversity of expertise, perspectives, and experiences of our community members. In this post we sit down with Dr. Helen Yannakoudakis, a winner of the Hateful Memes competition and an Assistant Professor at King’s College London, Visiting Researcher at the University of Cambridge, and co-founder and Chief Scientific Officer at Kinhub.
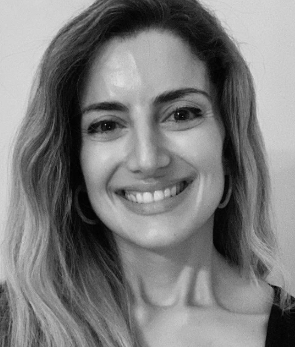
Name: Dr. Helen Yannakoudakis¶
Hometown: Athens, Greece¶
Tell us a little about yourself. Where do you live, what do you do professionally, what do you enjoy doing outside of work?
I’m an Assistant Professor at King’s College London, a Visiting Researcher at the University of Cambridge, and co-founder and Chief Scientific Officer at Kinhub. I work on machine learning for natural language processing, and I’m particularly interested in few-shot learning, lifelong learning, and societal and health applications such as abuse detection, misinformation, mental ill-health detection, and language assessment.
I live in London, but whenever we get the opportunity, my family and I love to travel, including to my home country of Greece, where we can top-up on vitamin D.
Outside of work, I love going to the theatre and the cinema (fan of Marvel and anything superhero-like), reading graphic novels (Logicomix is a must), and playing the piano (have been trying to master Nuvole Bianche for a while now!).
How did you get started in data science?
I was first introduced to the field of AI during my BSc studies in Computer Science at the Athens University of Economics and Business. My real journey, however, started when I moved to the UK to pursue my MPhil in Computer Speech, Text and Internet Technology at the University of Cambridge. I have been lucky enough to have had amazing mentors by my side, starting with my PhD supervisor, Ted Briscoe, who believed in me, and whose invaluable guidance and unwavering support have been instrumental in my development and shaping the researcher that I am today; and Paula Buttery, another inspirational leader in the field, from whom I have learnt a lot, and whose strong mentorship, generous time investment, and thought leadership have been pivotal to my growth as a researcher.
Data science is a broad field. What areas are you particularly interested in? Are there particular problems you’re interested in solving?
My research focuses on machine learning for natural language processing, with emphasis on few-shot learning and lifelong learning as well as societal and health applications. Few-shot learning and lifelong learning are key capabilities that separate current deep learning methods from more broadly applicable ones that do not hinge on the availability of large amounts of training data and do not suffer from catastrophic forgetting – therefore being fundamental components of the next generation of machine learning models.
Examples of my work, in both monolingual and cross-lingual settings, include FewShotTextGCN and Degree-of-Belief, as well as tasks such as document and text classification, dependency parsing, and word sense disambiguation.
What is a recent project you’ve worked on that you’re excited about or other interesting work you can share?
A recent piece of work that I’m particularly excited to share is FewShotTextGCN that focuses on few-shot learning on graphs. We specifically show how we can learn from scratch (i.e., without any language model pretraining) by effectively utilising the properties of word–document graphs, and outperform large pretrained language models on a range of languages using as little as 20 samples for training.
Other recent projects include our work on turning transformer-based sentence classifiers into zero-shot sequence labellers, and two new datasets: Ruddit, a dataset of English Reddit comments annotated with degrees of offensiveness; and the second version of the Teacher—Student Chatroom Corpus, a collection of written conversations captured during one-to-one online lessons between teachers and learners of English, annotated for grammatical errors as well as discourse and teaching-focused labels.
What motivated you to join a DrivenData competition or to continue participating in challenges?
These competitions offer the opportunity to contribute solutions to real-world challenges that have a positive and measurable societal impact. As a researcher, I also find particular value in the competitions’ ability to help bridge the gap between research and practice, paving the way to practical applications of cutting-edge research and continued innovation, which, in turn, is driving change and inspiring future generations of researchers to push the boundaries of this field and many others.
Is there a particular DrivenData challenge you’ve enjoyed working on?
A particularly interesting competition was the Hateful Memes Challenge that focused on the detection of harmful multimodal content. An increasingly common expression of online hate speech is multimodal in nature and comes in the form of memes. Designing systems that can automatically detect hateful content is of paramount importance if we are to mitigate its undesirable effects on the society at large. As memes convey a message using both images and text, effective detection depends on models that are capable of multimodal reasoning and joint visual and language understanding.
I worked with an amazing team of talented researchers from King’s College London and the University of Amsterdam – Phillip Lippe, Nithin Holla, Shantanu Chandra, Santhosh Rajamanickam, Georgios Antoniou, and Ekaterina Shutova – without whom our success in the challenge would not have been possible. We developed a framework of training algorithms and data sampling strategies that allowed us to better detect multimodal hate in unbalanced distributions and guide machine learning for memes that require subtle reasoning and understanding. The details of our approach can be found here and here.
Any good tools, posts, or projects from other developers that you appreciate or think the community might enjoy?
I am particularly excited about Chelsea Finn’s work and her amazing lectures on Deep Multi-Task and Meta Learning.
Anything else you want to share with the community? Is there anything the community could help you with?
Later this year, I will be hiring for postdocs and PhDs in Machine Learning and NLP. Further information to come – if interested, please stay tuned.
We will soon also be running a study with Samantha Capon on how to support parents with children suffering from mental health problems. We will be looking for participants in the near future. If interested, please watch this space.
Where can the community find you online?
Thanks to Dr. Helen Yannakoudakis (@heleny) for sharing your experiences and research with us! We are excited to feature more great community members. If you or someone you know would like to be in the Community Spotlight, let us know!