by
Christine Chung
U.S. PETs Prize Challenge¶
Privacy-enhancing technologies (PETs) have the potential to unlock more trustworthy innovation in data analysis and machine learning. Federated learning is one such technology that enables organizations to analyze sensitive data while providing improved privacy protections. These technologies could advance innovation and collaboration in new fields and help harness the power of data to tackle some of our most pressing societal challenges.
That’s why the U.S. and U.K. governments partnered to deliver a set of prize challenges to unleash the potential of these democracy-affirming technologies to make a positive impact. In particular, the challenge tackles two critical problems via separate data tracks: Data Track A will help with the identification of financial crime, while Data Track B will bolster pandemic responses.
The goal of the PETs Prize Challenge is to advance privacy-preserving federated learning solutions that provide end-to-end privacy and security protections while harnessing the potential of AI for overcoming significant global challenges. The challenge organizers hope to accelerate the development of efficient privacy-preserving federated learning solutions that leverage a combination of input and output privacy techniques to:
- Drive innovation in the technological development and application of novel privacy-enhancing technologies
- Deliver strong privacy guarantees against a set of common threats and privacy attacks
- Generate effective models to accomplish a set of predictive or analytical tasks that support the use cases
The challenge consists of three phases with a total prize pool of $800,000! Each phase invites participants to further test and apply their innovations in privacy-preserving federated learning.
|
Phase 1: Concept Papers¶
Phase 1 of the challenge was open to submissions from July 20th to September 19th, 2022. In this phase, competitors were tasked with writing a concept paper describing a privacy-preserving federated learning solution that tackled one or both of two tasks: financial crime prevention or pandemic forecasting.
The judges ranked the papers based on:
- Privacy: Provide robust privacy protection for the collaborating parties; Effectively prove or demonstrate the privacy guarantees offered by their solution, in a form that is comprehensible to data owners or regulators
- Accuracy: Minimize loss of overall accuracy in the model
- Efficiency: Minimize additional computational resources (including compute, memory, communication), as compared to a non-federated learning approach.
- Innovation: Show a high degree of novelty or innovation
- Generalizability: Demonstrate how their solution (or parts of it) could be applied or generalized to other use cases
- Usability: Consider how their solution could be applied in a production environment
For more details on the evaluation criteria, refer to the Problem Description.
Phase 1 saw an impressive amount of engagement, especially given the high-bar for participation. In total, we received 59 qualified submissions from 56 teams across all tracks! The papers included 19 addressing public health, 30 tackling financial crime, and 10 generalized solutions. The teams that submitted qualifying concept papers represented multiple sectors spanning academia and private industry, with individual participants including machine learning professionals, privacy researchers, public health experts, and more.
Judges reviewed a wide range of proposed privacy-enhancing technologies. The best solutions incorporate both "input privacy" (e.g., some kind of cryptography for confidentiality) and "output privacy" (e.g., differential privacy). Winning papers employed a wide range of methods including fully homomorphic encryption (FHE), secret sharing, differential privacy (DP), and secure multi-party computation (MPC). Competitors also utilized a wide range of models from tree-based algorithms like XGBoost to generative probabilistic models.
What's Next¶
Participants were required to complete a paper in Phase 1 in order to be eligible to compete in Phase 2, where they will develop their privacy-preserving federated learning concepts into working prototypes. This Solution Development phase will run through January, 2023.
Meanwhile, if you didn’t make it into the first two phases, don't worry! You still have an opportunity to participate in Phase 3: Red Teaming, where you'll prepare and test privacy attacks against Phase 2 solutions. Phase 3 registration is now open! Head over to the Phase 3 homepage to sign-up and compete for a share of $120,000. For more information on all the competition phases, check out the PETs Prize Challenge website.
Congratulations to all the participants in this phase! Meet the winners below and check out their approaches to advance federated learning in financial crime and pandemic forecasting!
Meet the winners: Phase 1¶
MusCAT¶
![]() |
![]() |
![]() |
![]() |
![]() |
![]() |
Place: 1st
Prize: $30,000
Team members: Hyunghoon Cho, David Froelicher, Denis Loginov, Seth Neel, David Wu, Yun William Yu
Hometown: Cambridge, MA; Boston, MA; Austin, TX; Toronto, ON (Canada)
Background:
We are a group of privacy-enhancing technology experts who are passionate about developing rigorous and practical privacy-preserving solutions to address real-world challenges:
- Hyunghoon Cho is a Schmidt Fellow at Broad Institute, where his research involves solving problems in the areas of biomedical privacy, genomics, and network biology with novel computational methods.
- David Froelicher is a Postdoctoral Researcher at MIT and Broad Institute with expertise in distributed systems, applied cryptography, and genomic privacy.
- Denis Loginov is a Principal Security Engineer at Broad Institute with the Data Sciences Platform with expertise in application security and cloud computing.
- Seth Neel is an Assistant Professor at Harvard Business School, where he works on theoretical foundations of private machine learning, including differential privacy, membership inference attacks, and data deletion.
- David Wu is an Assistant Professor at the University of Texas at Austin and works broadly on applied and theoretical cryptography.
- Yun William Yu is an Assistant Professor at University of Toronto, where he works on developing novel algorithms and theories for bioinformatics applications; his expertise also includes private federated queries on clinical databases and digital contact tracing.
What motivated you to participate?:
We viewed this challenge as an exciting opportunity to apply our expertise in privacy-enhancing technologies to help address a timely and meaningful real-world problem. We also believed that the range of relevant backgrounds our team covers, which include both theoretical and applied research in security, privacy and cryptography, algorithms for biomedicine, as well as cloud-based software engineering and deployment, would uniquely enable us to develop an effective solution for the challenge.
Summary of approach:
Our solution introduces MusCAT, a multi-scale federated system for privacy-preserving pandemic risk prediction. For accurate predictions, such a system needs to leverage a large amount of personal information, including one’s infection status, activities, and social interactions. MusCAT jointly analyzes these private data held by multiple federation units with formal privacy guarantees. We leverage the key insight that predictive information can be divided into components operating at different scales of the problem, such as individual contacts, shared locations, and population-level risks. These components are individually learned using a combination of privacy-enhancing technologies to best optimize the tradeoff between privacy and model accuracy.
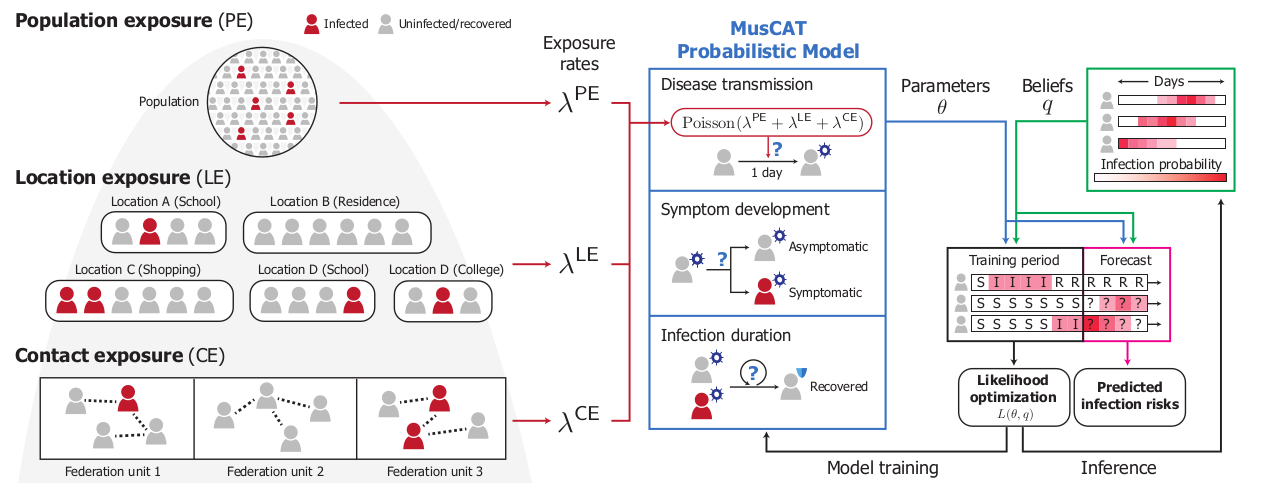
A graphical overview of MusCAT: A multi-scale, hybrid federated system for privacy-preserving epidemic surveillance and risk prediction..
IBM Research¶
![]() |
![]() |
![]() |
![]() |
![]() |
![]() |
![]() |
![]() |
![]() |
![]() |
![]() |
![]() |
![]() |
![]() |
![]() |
![]() |
![]() |
Place: 2nd
Prize: $15,000
Team members: Heiko Ludwig, Nathalie Baracaldo Angel, Swanand Kadhe, Yi Zhou, Alan King, Keith Houck, Ryo Kawahara, Mikio Takeuchi, Hayim Shaul, Nir Drucker, Naoise Holohan, Ambrish Rawat, Mark Purcell, Lam Nguyen, Eyal Kushnir, Giulio Zizzo, Ehsan Degan
Hometown: San Francisco, CA; San Jose, CA; Yorktown Heights, NY; Rye, NY; Ossining, New York; Tokyo, Japan; Yokohama, Japan; Kfar-Saba, Israel; Haifa, Israel; Dublin, Ireland
Affiliation: IBM Research
Background:
The IBM Research team is composed of members working in different labs around the world, working on various topics of the security and privacy of AI. This includes a team with a background in homomorphic encryption, differential privacy, privacy-preserving machine learning, federated learning, robustness against adversarial attacks, and graph algorithms. Collectively, this team has a large body of publications in the field and has worked on publicly available software such as IBM FL, HELayers, the Adversarial Robustness Toolbox, and diffprivlib.
What motivated you to participate?:
Combining the benefits of machine learning with the responsible and accountable use of data is imperative, both for individuals but also for companies providing important services. Money laundering is not a victimless crime but enables the drug trade, human trafficking and other crimes with large, terrible effects on society and individuals. We need good mechanisms to uncover money laundering while still preserving individuals’ privacy in a free society. IBM Research has worked on this topic for a number of years and this competition provides a great way to further the effort in a friendly competition. However, it’s also good business, both for financial institutions and their suppliers of key information technology, such as IBM.
Summary of approach:
It is not possible to use existing Federated Learning approaches in a plug-and-play manner when data is partitioned both vertically and horizontally. Our solution combines cryptography, differential privacy, and randomization techniques applied to create a novel cryptographic protocol that overcomes the necessity to explicitly perform record alignment. Our approach generates decision tree ensembles to balance privacy and accuracy during training and prevent inference threats at model deployment time. This approach enables formal privacy guarantees on training data while banks and SWIFT train collaboratively and protects output privacy during inference. The entire stack is designed to be efficient and scalable.
Secret Computers¶
Place: 3rd
Prize: $10,000
Team members: Kevin McCarthy, Dr. Sergiu Carpov, Dr. Marius Vuille, Dr. Dimitar Jetchev, Dr. Mariya Georgieva Belorgey, Manuel Capel
Hometown: New York, NY
Affiliation: Inpher, Inc.
Social Media: Twitter; LinkedIn
Background:
Inpher, Inc. is a global Secret Computing© company that powers privacy-preserving machine learning and analytics to unlock the value of sensitive, siloed data and enable secure collaboration across teams, organizations and nations. Data scientists leverage Inpher’s XOR Platform to train and run ML models on deeper and more diverse data while it is encrypted to improve model performance with mathematically guaranteed data privacy and residency.
Global enterprises and government agencies use XOR in multiple vertical applications across finance, healthcare and national security. Inpher’s team of recognized leaders in the fields of Multiparty Computation (MPC), Fully Homomorphic Encryption (FHE), and Federated Learning (FL) continue to deliver the fastest, high-precision privacy-preserving computing capabilities.
What motivated you to participate?:
Our mission is to drive societal benefits with data-driven insights while safeguarding privacy and individual liberties; we believe that in the domain of fraud detection and counter financial crime, it takes a network to stop a crime network. Through the proper use of Privacy Enhanced Technologies (PETs), the Financial Ecosystem has the power to leverage collective intelligence to mitigate financial crime and its illicit implications. Inpher has been an advocate for PET applications within counter financial crime efforts since the company's inception, participating in numerous regulatory techsprints and hackathons as well as ongoing commercial applications. The U.S.-U.K. PETs Challenge elevates awareness about the opportunity while closing the gap between research and reality to realize the societal benefit of these technologies.
Summary of approach:
Inpher proposes a scalable, privacy-preserving federated architecture for high-precision statistical analysis and ML utilizing our commercial XOR Platform. For this challenge, XOR enables and orchestrates privacy-preserving capabilities using Private Set Intersection and Secure Multiparty Computation for distributed Gradient Boosting and Logistic Regression models to predict anomalies. The solution provides cryptographic security proofs using a novel framework that is optimized for ML applications like financial anomaly detection which requires high numerical precision without compromising data privacy and efficiency.
More on Inpher’s approach and the XOR Platform:
- Solution Brief: Reimagining Fraud Detection with Collaborative, Privacy Enhanced Technologies
- Explainer Video: Youtube - How Secret Computing® Works
- Developer Documentation: XOR Product Documentation & Free Trial
Don't forget to get your submissions in for Phase 2 by the due date on January 24, 2023. If you aren’t competing in the first two phases, you can still sign up for Phase 3: Red Teaming, where you'll put Phase 2 solutions to the test. Head over to the U.S. competition website for your chance to tackle pressing challenges in privacy-enhancing technologies and win a share of $800,000 in prizes. Good luck!
Thanks to all the participants and to our winners! Special thanks to the National Institute for Standards and Technology (NIST) and the National Science Foundation (NSF) for enabling this fascinating challenge, the UVA Biocomplexity Institute and SWIFT for providing the datasets, and the judges and reviewers for their time and input!