This week, we got to hear from Dr. Jason Hostetter (@jhostetter) a clinical radiologist and member of our Technical Advisory Panel who has been instrumental to the design of the Concept to Clinic challenge.
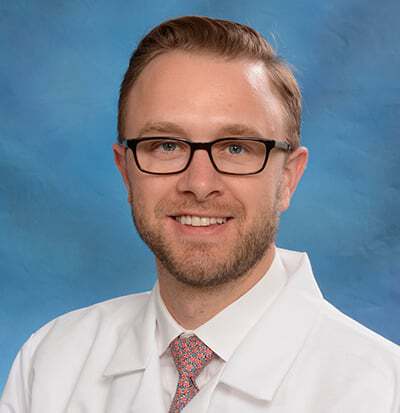
Hi Jason, who are you and what is your background in radiology and software?¶
I am a clinical fellow at Johns Hopkins University, in the department of Neuroradiology, and formerly a radiology resident at the University of Maryland. I have been practicing radiology as a resident and fellow for four and a half years in Baltimore.
I began learning how to program back in college and worked as a full-time developer briefly after college, before starting medical school. Over the years of medical training I kept an interest in software and data management, and this became part of my interest in radiology. We have a huge amount of digital data, but few good ways to mine it and compound its value.
Why did you choose to participate as an advisor for this project?¶
I believe in the power of like-minded groups of people, and have personally benefitted from hundreds of open source projects. I loved the idea of leveraging the power of programmers across the world to tackle one of the biggest needs in medical imaging right now, data collection. Machine learning and AI is exploding right now in the world of medical imaging, but we have precious few reliable datasets to train algorithms, and fewer still good ways to collect it.
What do you see as the biggest areas where better uses of machine learning can help radiologists with their work?¶
Automating or streamlining mundane but important tasks. For instance, finding lung nodules and tracking their size over time. Same thing with lymph nodes or tumors in cancer follow-up studies. These tasks are tedious and time consuming for humans, but play a very important role in treatment. They also tend to be quite routine and with well-described parameters for reporting, which is perfect for a computer algorithm.
Another area is integrating and visualizing many types of data, for instance, texture analysis, dual-energy CT data, MRI spectroscopy, perfusion, etc. There are many new and emerging imaging technologies, some of which don't even have a standard method of visualization. These data may be able to be analyzed and integrated more effectively by computers than by humans. These examples only scratch the surface of how machine learning can help us make our jobs faster, more accurate, and safer.
What are the benefits of an open source challenge like this? In what ways is this project useful?¶
Open source can not only drive the production of great software, it also brings together a community of people who believe in the same end goal. Newcomers can be brought into the conversation and learn about what is being created. Medical imaging is a relatively small world compared to the wider world of software development. This project helps skilled programmers see what problems our industry is struggling with, and gives them an opportunity to dive in to our world.
With the ultimate goal of better health care for real people, I hope contributors can see the potential impact of what they produce. It's about more than shipping a product to market, or pleasing investors. That higher goal is what sets a project like this apart.