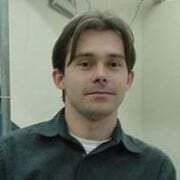
We got a chance to hear from Gilberto Titericz Jr., our Countable Care 1st place finisher. He answered some of our questions about himself, the competition, and data science in general.
Congratulations on winning the Countable Care competition! Who are you and what do you do?¶
I'm an electronics engineer with a M.S. in telecommunications. In the past 16 years I've been working as an engineer for big multinationals like Siemens and Nokia, and later as an automation engineer for Petrobras Brasil.
What first got you interested in data science?¶
In 2008 I started to learn by myself many machine learning techniques, first trying to improve personal stock market gains. In 2012 I started to compete in international Machine Learning competitions and have learned a lot more since then.
How long have you been at Petrobras, what kind of problems do you work on, and what were you doing before?¶
I've been working for Petrobras since 2008. My current working day includes analyzing and optimizing automation logic of heavy equipment.
What brought you to DrivenData or this problem in particular?¶
I try to join all data science competitions that I can. When I placed 3rd in DrivenData's previous competition, I just waited for a new one and when "Countable Care" was released I rapidly joined it and started coding. The dataset is small but very good to work on.
Can you describe your initial approach to this problem? When you’re first exploring a new data set, what are your personal habits for getting familiar with the data?¶
My approach is based on an ensemble of models. To make it possible, I trained a lot of models, with different hyperparameters, different numbers of features, and different learning techniques. That was the first level of learning.
All training was made using a 4 fold cross validation technique, where it generates two predictions sets: one cross validated train set and one test set for each model. All those models are then ensembled using a second level of learning, training with all the cross validated train sets (meta features), and then the final model is applied in the predicted test sets.
That approach was chosen because the dataset is composed of 1,379 features, most of it categorical, and training instances are not big enough to do a reliable feature selection. As the predictive power of the dataset comes mainly from the categorical features whose features don't have many levels, I decided to quit simple feature selection and use the ensemble technique to better explore feature interaction between all features and different learning techniques. My final ensemble was composed of 9 models:
- 1 Random Forest
- 6 Xgboost
- 2 Logistic Regression
When first exploring a new dataset I always visualize the data, try to identify categorical and numeric features, identify correlation of features with each other and target, then run a single cross-validated training to observe the performance that dataset can achieve.
Was there anything that surprised you in working on this particular problem? Anything that worked particularly well (or not well) that you weren’t necessarily expecting?¶
Ensemble of model at a second level worked very well at this competition. But one thing that did not work very well was feature selection. Probably due to the size of the dataset, but selecting good features just led to overfitting.
What programming languages do you like to use for data science tasks? What are your thoughts on the current and future scientific computing ecosystem?¶
I use R 70% of the time and Python 30%. Machine learning tools, services and products are each day more in demand in the world market. So there is a great future for Data Science, because the scientific environment will grow even bigger in the next few years.
And a company with a talented Data Scientist equipped with high end software and hardware can be a secret weapon.
What machine learning research interests you right now? Have you read any good papers lately?¶
I like ensembling and feature selection techniques. Also I started to learn convolutional networks and read some articles about that. But it needs good hardware, so buying a PC with a good GPU is my next step.
It seems like data science in particular has an incredible number of self-directed learners and people with nontraditional career paths. Do you have any recommendations for people trying to break into the field and learn the sort of applied skills you used in this competition?¶
There is no end in the Data Science learning curve. You always are learning something new. I found joining competitions are the best way to build strong knowledge in that field. Mostly because it obligates you to read papers, articles and forums about a specific problem. Also it makes you think about new possibilities and makes you try different aproaches to the problems. So...Join a competition and learn more and more!
Now some just for fun questions about your setup (hat tip to usesthis.com!) -- in your daily personal and working life, what hardware do you use? Would you mind sharing a picture of your hardware setup/desk?¶
My home hardware is a mid-end Toshiba laptop equipped with a Core i7 generation 3, a 120 GB SSD main disk, and 24 GB of RAM.
My work hardware is a low-end Core i5 with 4GB of RAM :-((
And what software?¶
My laptop smoothly runs Ubuntu 14.04 LTS, R Studio Server and Spyder Python IDE. Those are the basics, and enough.
Would you mind sharing a screenshot of your desktop?¶
Here it is:
A big thanks goes out to Gilberto from the DrivenData team for taking the time to chat. Feel free to discuss this interview in the DrivenData community forum, and stay tuned for the conclusion of our Keeping it Fresh competition!